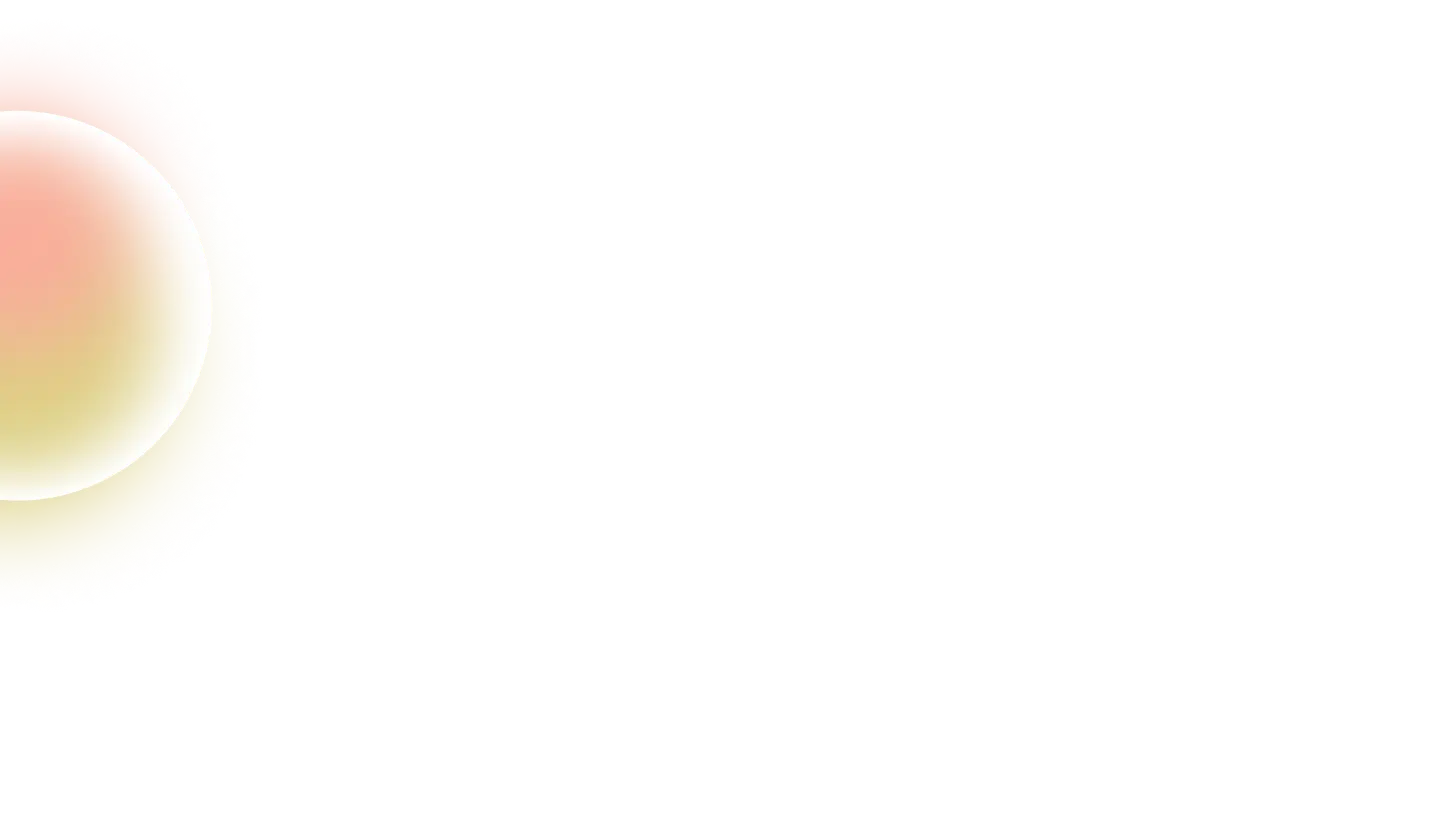
The Hidden Roadblocks to Personalisation (And How to Fix Them)
Marketing personalisation can unlock huge potential, but many businesses are running into hidden roadblocks. In this post, we explore the key challenges slowing down your personalisation efforts, from poor data accessibility to a lack of real-time insights. We’ll also discuss actionable steps to fix these problems and get your personalisation strategy back on track.
Many businesses invest heavily in marketing personalisation with high expectations—more engagement, stronger customer loyalty, and increased revenue. Yet, too often, these efforts stall at basic segmentation or generic recommendations, failing to deliver the impact promised.
The issue isn’t a lack of ambition. It’s a cycle of budget constraints, poor data integration, and organisational silos that prevent true personalised customer experiences. This blog explores the most common roadblocks to personalisation in digital marketing and provides a clear roadmap to overcoming them.
The Vicious Cycle of Marketing Personalisation Failure
Personalisation challenges aren’t isolated issues. Businesses often find themselves trapped in a self-reinforcing cycle, where each problem compounds the next, making it harder to break free.
Budget Constraints
Effective AI personalisation isn’t just about purchasing the right tools—it requires ongoing investment in data infrastructure, integration, and expertise. Many businesses opt for quick fixes due to limited budgets, leading to underwhelming results.
Customer Data Integration Challenges
The root issue isn’t a lack of data—it’s the inability to access and integrate it effectively. Different teams, such as marketing, CRM, and IT, often operate in silos, leading to fragmented and incomplete customer segmentation. Without a unified view, personalisation efforts fall short.
Poor Results Lead to Stalled Investment
When personalisation doesn’t drive tangible improvements, businesses struggle to justify further investment. The issue? Many companies fail to measure success correctly. If you’re only tracking click-through rates without linking personalisation efforts to revenue or customer retention, it’s difficult to prove value.
The team ran the campaign using Plinc’s own Category Affinity Model, which uses daily data signals to predict what product areas each customer is most likely to care about. But the broader point here goes beyond any one tool: smarter targeting doesn’t have to mean over-engineering. When teams move from static segmentation to dynamic, behaviour-led signals, both CRM and Trade win.
The Root Cause – Data Accessibility & Integration
While AI can enhance personalised customer experiences, it cannot compensate for poor data quality. Without real-time, structured insights, even the most sophisticated personalisation strategies will fail. Businesses need a well-integrated data ecosystem that ensures marketing, CRM, and customer service teams can access and leverage the same high-quality, up-to-date information to drive effective campaigns.
Lack of Access to Data
Many marketing teams struggle with limited visibility into customer data due to restrictive permissions or outdated systems that create barriers to access. Without proper access, marketers are left relying on incomplete datasets, which undermines their ability to deliver truly personalised experiences.
Ensuring teams have the necessary permissions and implementing centralised data management systems can help businesses eliminate these accessibility challenges.
Siloed Data Across Departments
A major roadblock to customer segmentation and effective personalisation is the lack of seamless data sharing between key departments. When marketing, CRM, and customer service teams work with disconnected datasets, their understanding of customer needs remains fragmented, leading to inconsistent experiences.
Businesses must break down these silos by integrating data sources across departments, ensuring every team operates with a unified customer view that enables more accurate personalisation efforts.
Outdated Data
The success of personalisation in digital marketing relies on real-time insights, yet many businesses rely on delayed or static data that quickly becomes irrelevant. Outdated data leads to recommendations that fail to reflect customer behaviour, such as promoting products a customer has already purchased or sending offers that no longer align with their interests.
To avoid this, businesses must invest in automated data refresh systems that ensure customer insights are always up to date and can dynamically inform personalisation strategies.
Signs Your Personalisation Strategy Has a Problem
Not sure if your personalisation in digital marketing is working? Here are three red flags to look out for:
Your Campaigns Rely on Segmentation Instead of Individualisation
Grouping customers by demographics or purchase history is not true personalisation. Predictive, real-time personalisation goes beyond static segments to anticipate intent and serve dynamic experiences.
Your Personalisation Feels Repetitive or Generic
If customers keep seeing the same recommendations, personalisation fatigue sets in. Strategies must evolve based on real-time behaviours, not just past actions.
Your Metrics Aren’t Improving
If engagement, conversion rates, or revenue aren’t increasing, your personalisation efforts are missing the mark. Success should be measured beyond click-through rates, focusing on long-term retention and customer lifetime value.
How to Break the Cycle
To move beyond the common roadblocks, businesses must take a structured, data-first approach to personalisation. The key to success lies in ensuring that all personalisation efforts are aligned with measurable business objectives, built on high-quality data, and supported by a cohesive strategy that integrates teams across the organisation.
Set Clear Business Goals
A successful personalised customer experience strategy must begin with clearly defined business objectives. Personalisation should be more than just an engagement tool—it should directly contribute to measurable outcomes such as revenue uplift, customer lifetime value (CLV), and retention rates. Without well-defined KPIs, it becomes difficult to determine whether personalisation initiatives are actually delivering value.
Setting clear goals from the outset also helps in securing buy-in from leadership teams. When business executives can see a direct link between personalisation efforts and financial performance, they are far more likely to support ongoing investments in data and technology. Furthermore, well-defined goals ensure that personalisation strategies remain focused and scalable, rather than fragmented and reactive.
Prioritise Data Quality
No matter how advanced your AI-model is, data-driven personalisation’s effectiveness is entirely dependent on the quality of the data it processes. If your customer data is outdated, incomplete, or stored in disconnected systems, your AI will be working with an unreliable foundation—ultimately leading to inaccurate recommendations and missed opportunities.
To ensure optimal data quality, businesses should begin with a comprehensive data audit. This involves identifying inconsistencies, duplicate records, and gaps in data collection. By assessing the health of existing data assets, organisations can take corrective action before deploying AI-driven strategies.
Another critical step is the implementation of real-time data updates. Many businesses fall into the trap of relying on batch-processed insights, which can quickly become outdated in fast-moving industries such as retail or finance. By leveraging automation and AI-powered data integration tools, businesses can ensure that customer insights remain current and actionable.
Finally, automated customer insights should be integrated across all relevant platforms. A unified data ecosystem enables marketing teams to access the most up-to-date customer profiles, ensuring that personalisation efforts are both relevant and responsive. This also allows for a seamless omnichannel experience, where customers receive consistent and timely interactions across email, social media, and web platforms.
Foster Cross-Functional Collaboration
Personalisation is not solely a marketing initiative—it requires the collaboration of multiple departments, including IT, CRM, and customer service teams. Siloed operations prevent businesses from fully leveraging their data, leading to inconsistent and ineffective customer experiences.
To break down these barriers, businesses should establish cross-functional teams dedicated to personalisation initiatives. These teams should include stakeholders from different areas of the organisation, ensuring that insights are shared and strategies are aligned. Additionally, businesses should invest in tools that facilitate seamless data sharing between departments, eliminating bottlenecks that prevent personalisation efforts from reaching their full potential.
Fixing Customer Data: Case Studies
Many businesses have successfully overcome data challenges to power their AI personalisation strategies. Here are three examples of brands that transformed their customer data infrastructure by working with Plinc, enhancing their personalisation strategies and successfully increasing customer engagement.
Halfords: Centralising Data for Omnichannel Personalisation
Halfords, a leading omnichannel retailer, needed a more cohesive approach to personalisation across online and in-store interactions.
By unifying data from multiple sources—including transactional, behavioural, online, and offline touchpoints—they built a comprehensive customer profile.
This integration allowed them to capture real-time intent signals, enabling highly targeted product recommendations and consistent, relevant messaging across customer interactions, even at the point of sale.
M&S International: Optimising Promotions with Predictive Analytics
M&S International sought to refine its promotional strategies by leveraging high-quality, predictive customer insights.
By consolidating data from various sources—including customer profiles, past transactions, and online behaviours—they created a single, accessible customer view.
Using Plinc’s Future Value Model, they could predict customer value based on past interactions, allowing them to tailor promotions more effectively, optimise marketing spend, and drive incremental revenue.
Crew Clothing: Enabling Self-Serve, Real-Time Insights
Crew Clothing wanted a real-time view of customer behaviour, especially during peak trading periods such as Black Friday. To achieve this, they developed a continuously updated Single Customer View, integrating data from multiple sources into a single platform accessible to key stakeholders.
With intuitive dashboards providing immediate insights, their teams could make faster, data-driven decisions, optimising campaigns and business strategies based on live customer behaviour.
These case studies demonstrate that businesses that prioritise data accessibility, integration, and real-time insights can unlock the full potential of personalisation in digital marketing. By addressing foundational data challenges, brands can improve engagement, increase conversions, and drive long-term customer loyalty.
To learn more about these real-world data success stories, explore Plinc’s case studies.
Closing Thoughts
Personalisation success is not just about technology—it’s about data, integration, and strategy. Businesses that invest in data accessibility, cross-team alignment, and predictive insights will unlock true, scalable personalisation.
Key Takeaways:
Fix data quality and ensure real-time updates.
Align teams to break down data silos.
Focus on measurable business impact, not just AI adoption.
Is your personalisation strategy delivering real results? Take a deep dive into your current approach and explore how Plinc’s integrated platform can help improve your data accuracy with real-time insights.
Check out our latest report on predictive personalisation insights to future-proof your strategy.
Blog posts
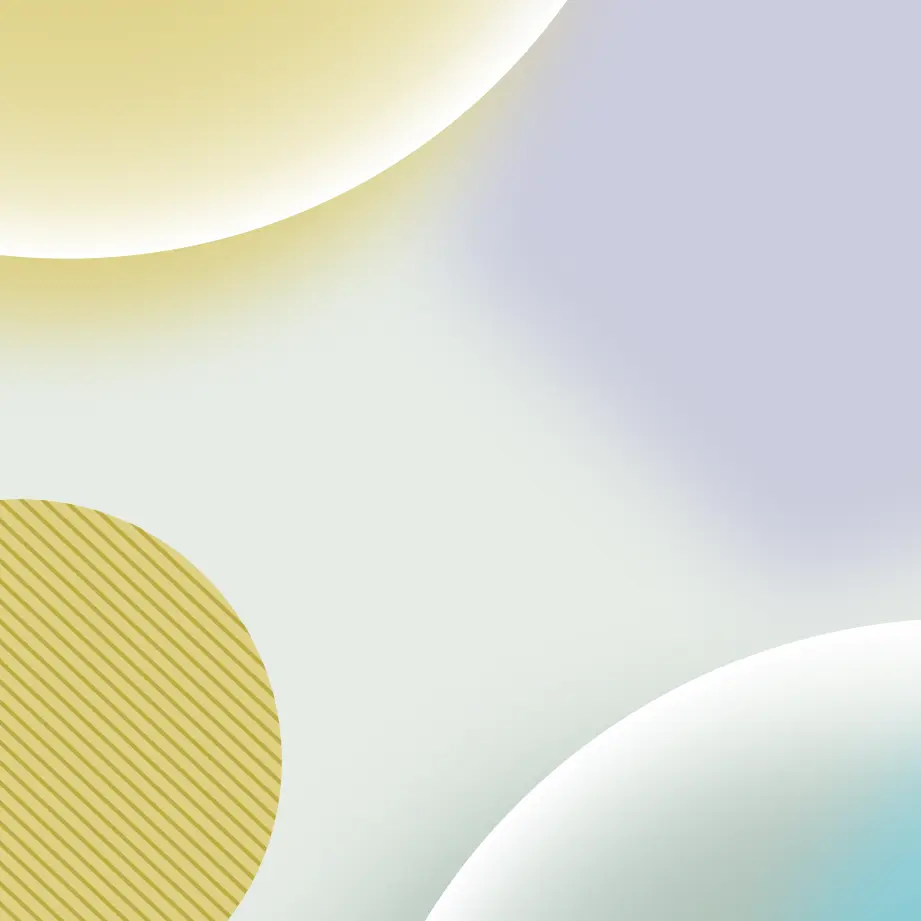
See Beneath the Surface: Turning Segmentation into Strategic Intelligence
Segmentation isn’t just for targeting. When used to surface behavioural insight, it reveals where value is created, what predicts loyalty, and how CRM can…
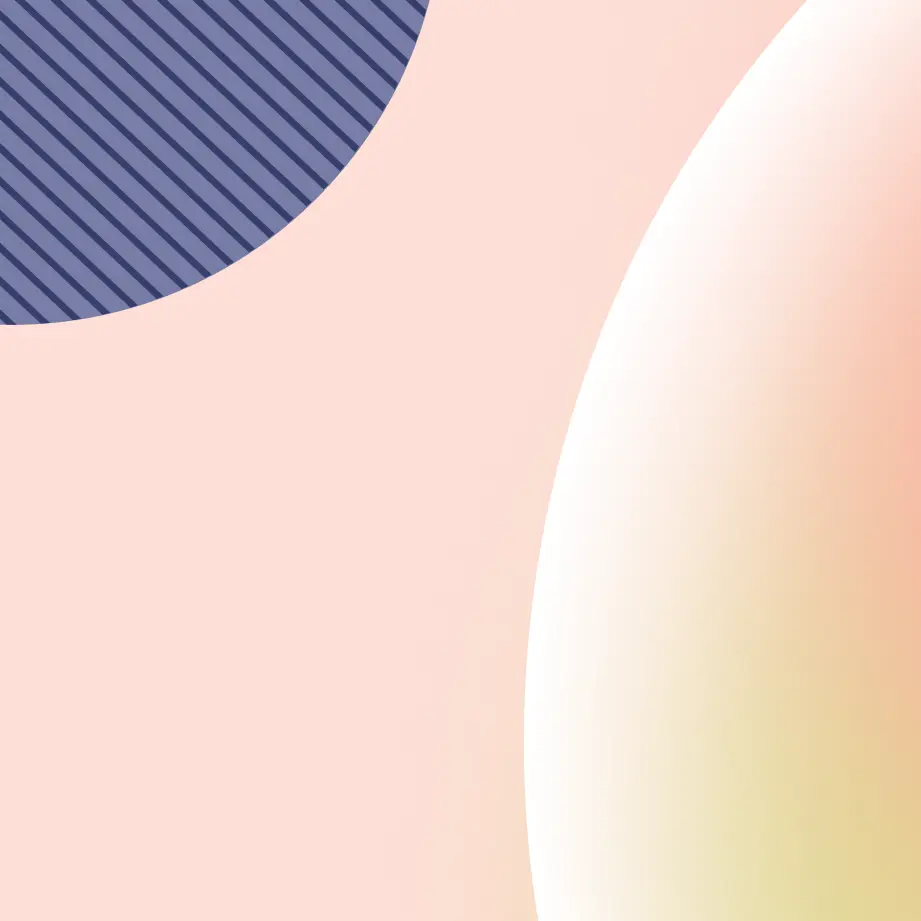
Become a Customer Economist: Why CRM Should Speak in Value, Not Volume
CRM is often rich in insight but fails to speak the business’s language. Reframing performance in terms of customer value is key to influencing strategy…
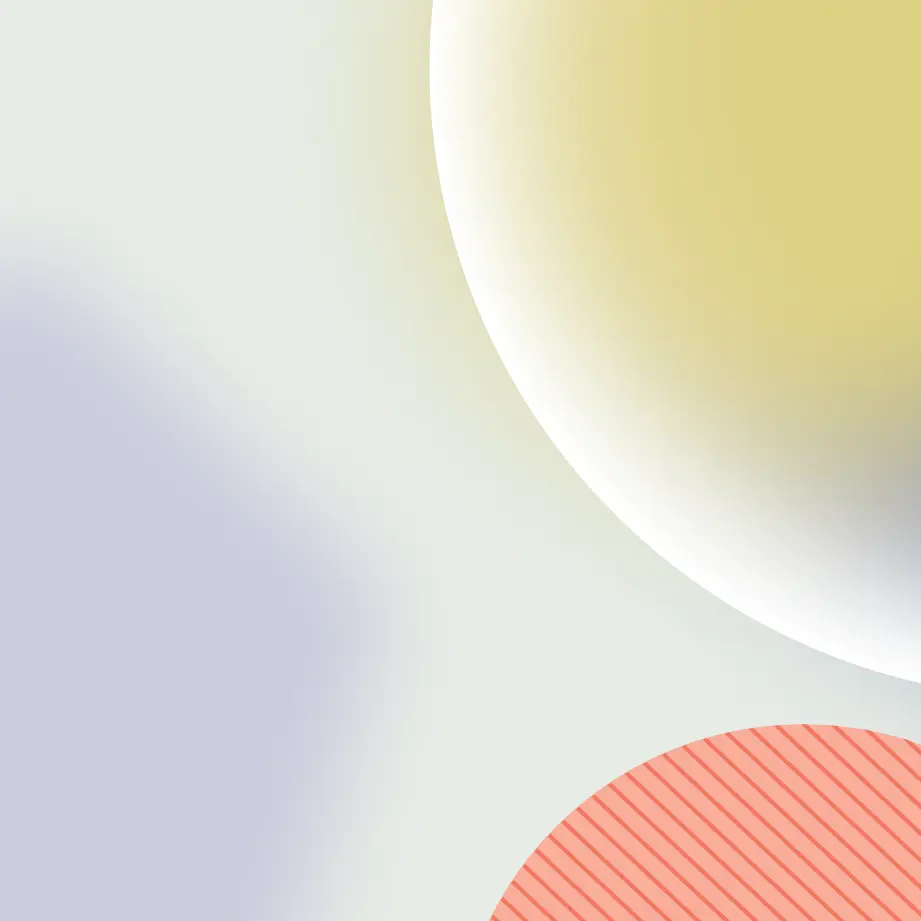
From Batch to Precision: Winning the Relevance War Without Burning Out Your Base
Batch campaigns create CRM fatigue fast. Shifting to precision targeting protects customer value, boosts engagement efficiency, and builds sustainable…
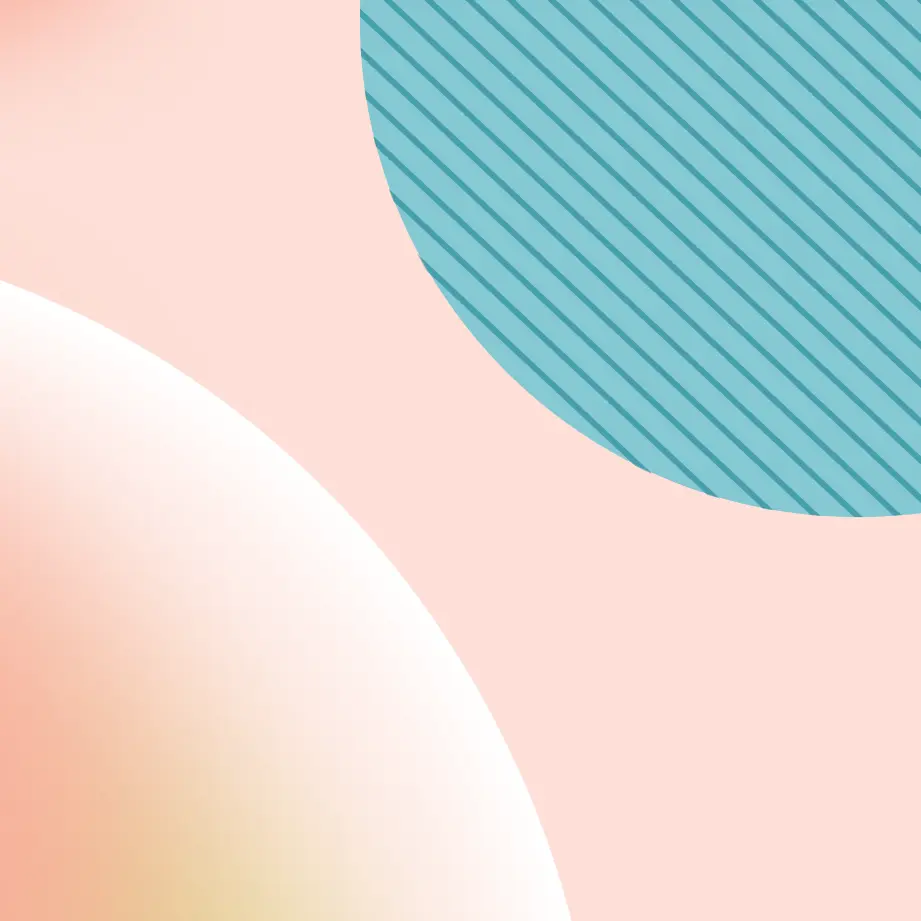
Prove It or Lose It: Why CRM Needs to Speak in Revenue, Not Reach
CRM teams are under pressure to prove commercial impact. Without uplift measurement and control groups, incremental revenue stays invisible and influence…
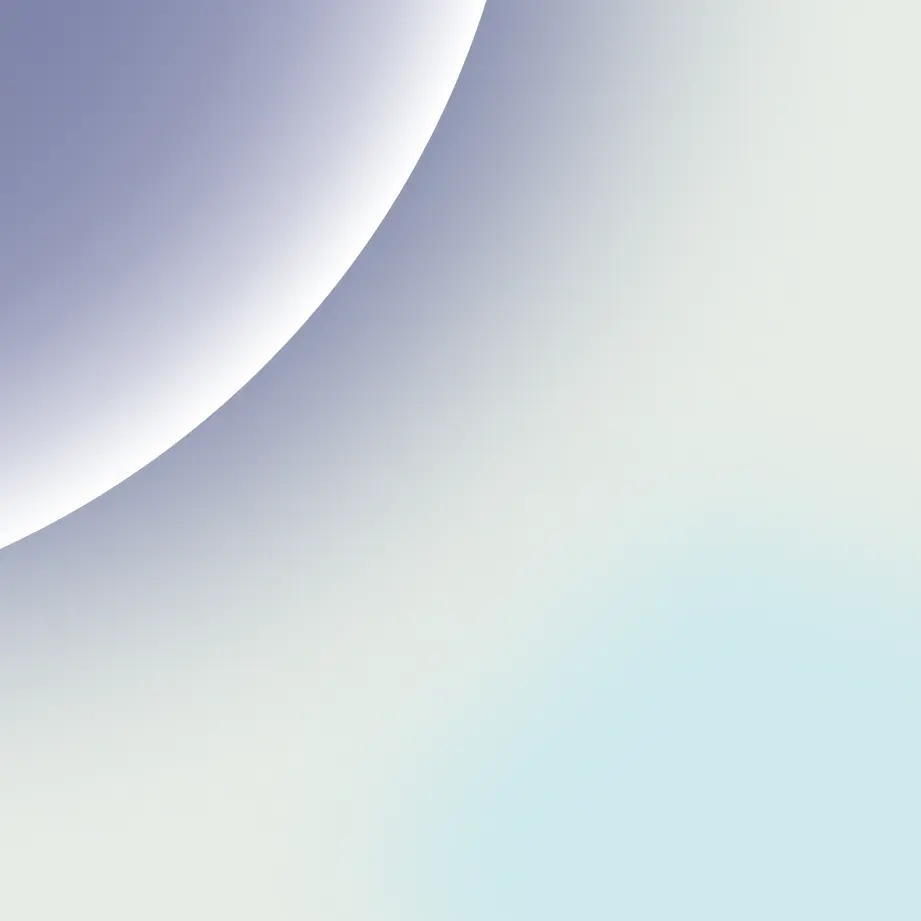
Sound the Alarm: Why CRM’s Data Problem Is a Strategic Problem
Inaccessible data continues to limit the impact of CRM. Yet when it’s reframed as a commercial issue rather than a technical one, it opens the door to…
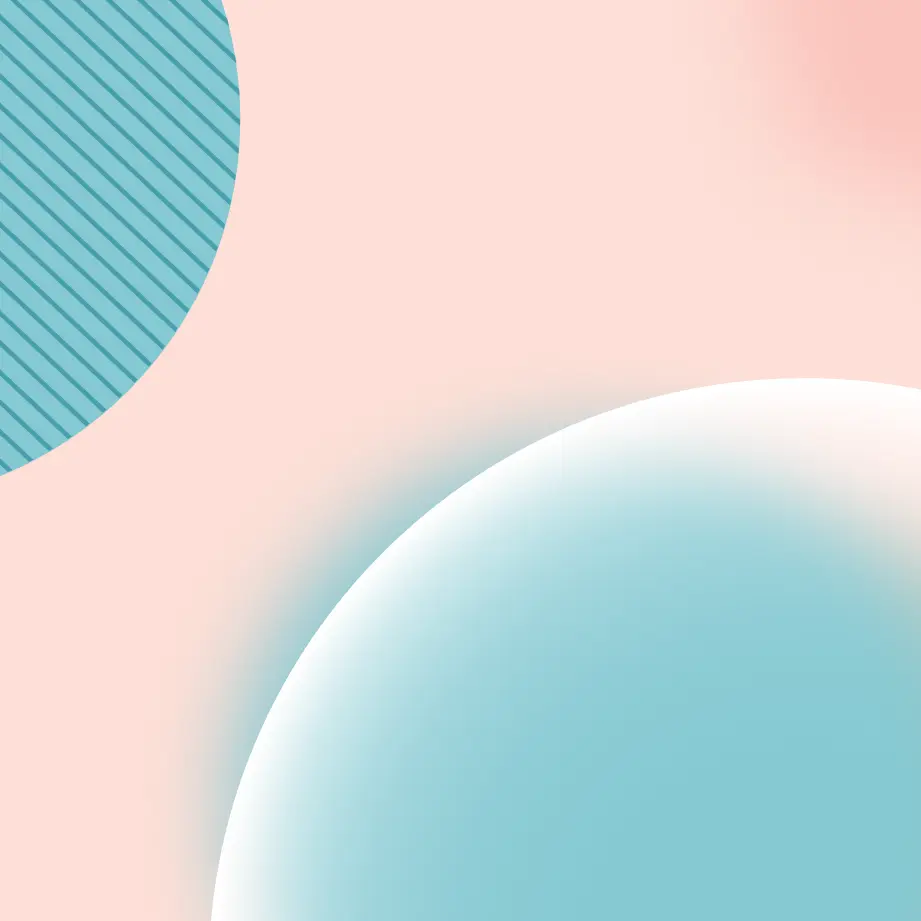
From Execution to Influence: Why It’s Time for CRM to Take the Lead
Introducing Plinc’s Strategic CRM Playbook: From Execution to Influence. Discover why CRM is poised to lead with insight, value, and influence in today’s…