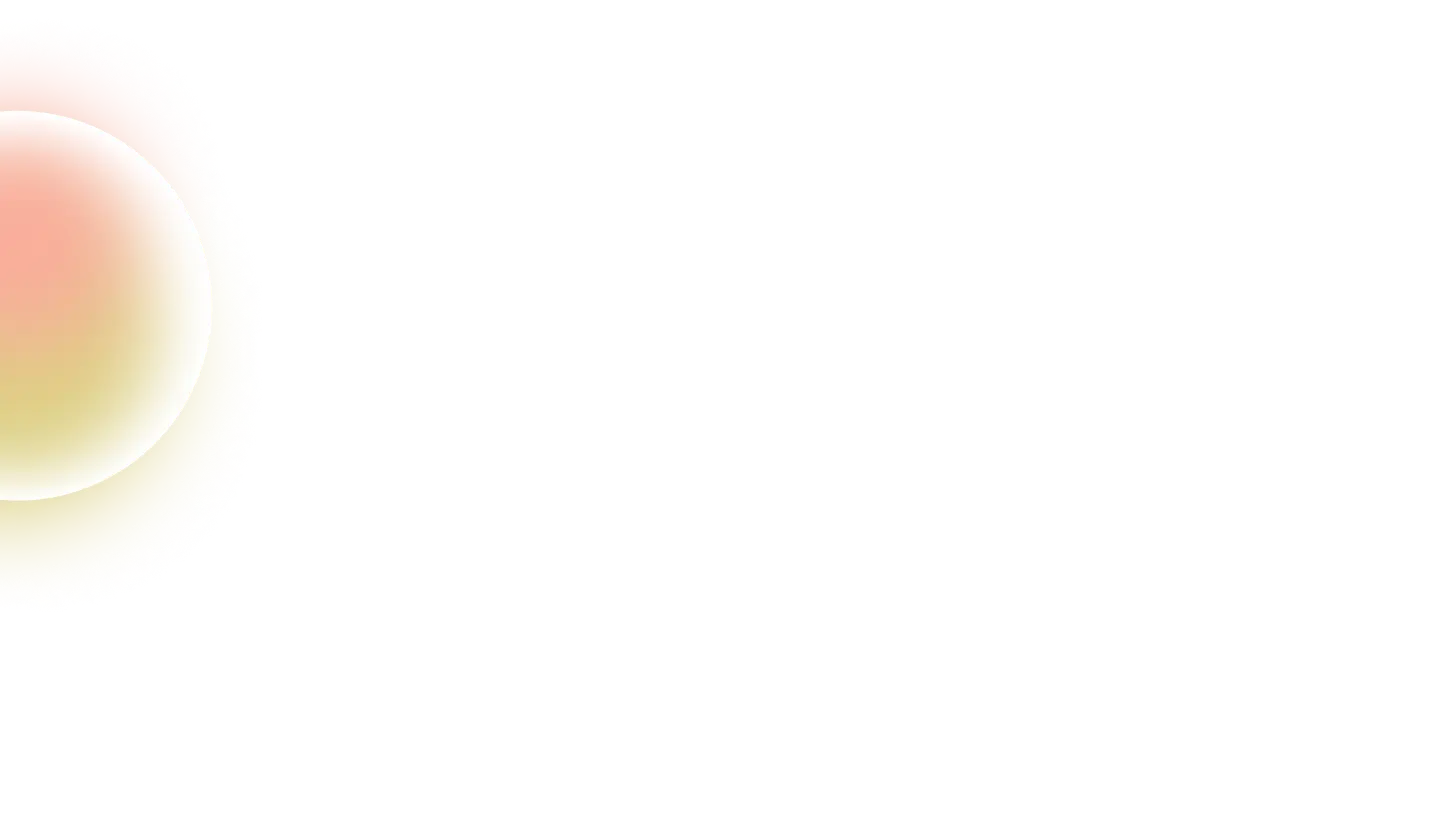
AI Won’t Fix Bad Data: The Truth About Machine Learning and Personalisation
Investing in AI for personalisation without fixing your data is like building a house on a shaky foundation. In this blog, we explore why AI alone won’t solve your personalisation challenges and how data quality and integration are essential for success. Discover why getting your data right is the first step to unlocking AI’s full potential in personalisation.
Artificial Intelligence (AI) is revolutionising marketing personalisation, offering brands the ability to deliver hyper-relevant customer experiences at scale. But there’s a catch. Many businesses assume that AI alone will transform their marketing strategies—only to be disappointed when their personalisation efforts fall flat.
The hard truth? AI can’t fix bad data. No matter how sophisticated the model, if the foundation isn’t right, AI-driven personalisation will fail. Instead of delighting customers with timely, relevant content, businesses risk delivering irrelevant, outdated, or even embarrassing experiences.
The key to truly personalised customer experiences doesn’t lie in AI alone. It hinges on data quality, integration, and strategic alignment. Before investing in AI-driven personalisation, businesses must ensure they have the right data infrastructure in place.
Why AI Alone Can’t Deliver Effective Personalisation
AI relies on structured, real-time, and integrated data to make accurate predictions. Without this, even the most advanced AI models will produce unreliable, inconsistent results. The reality is that most businesses struggle with fragmented, siloed, or outdated data, making AI ineffective from the outset.
The principle is simple: garbage in, garbage out. If your input data is flawed, your AI-driven personalisation strategy will be, too.
“We see businesses rushing to invest in AI-driven personalisation without addressing the root problem—data quality and integration. AI can’t fix bad data. It’s like trying to build a high-speed train on broken tracks.”
– Stuart Russell, Plinc
The Common Pitfalls of AI in Personalisation
Lack of Historical Data
AI personalisation depends on a deep history of customer behaviour to predict future actions with accuracy. However, many businesses lack long-term behavioural insights, either because they haven’t collected sufficient data or because their existing data infrastructure doesn’t retain historical interactions.
Without this depth, AI is forced to rely on limited or short-term patterns, resulting in generic, weak, or inaccurate predictions that fail to reflect customers’ true preferences and intentions.
Siloed Data Sets
One of the most significant challenges in AI-driven personalisation is the fragmentation of customer data across different departments. Marketing, sales, customer service, and product teams often maintain separate data systems that don’t communicate with each other, preventing the creation of a unified customer profile.
Without a seamless data infrastructure, AI models work with incomplete customer insights, leading to disjointed, inconsistent experiences where customers receive irrelevant offers, duplicated messages, or poorly timed recommendations that don’t align with their recent interactions.
Delayed Data Processing
Personalisation in digital marketing is most effective when it happens in real-time. AI needs to analyse and act on customer data as it evolves, responding dynamically to recent interactions and intent signals. However, many businesses rely on batch data processing, where insights are updated periodically rather than continuously.
This delay means businesses may send recommendations or promotions that no longer align with a customer’s preferences, leading to missed engagement opportunities and a loss of customer trust.
How to Make AI Work for Personalisation
Fix Data First
Before implementing AI, businesses must ensure that their data ecosystem is structured, real-time, and well-integrated. The first step is conducting a comprehensive data audit to identify gaps, inconsistencies, and silos. This involves assessing the quality and completeness of customer profiles, ensuring that all relevant data—transactional, behavioural, demographic, and engagement history—is captured and stored in an accessible format.
By cleaning and enriching customer data, businesses provide AI models with a solid foundation, allowing them to generate accurate, meaningful insights that enhance personalisation efforts.
Use AI to Enhance, Not Replace, Strategy
AI should be a powerful tool in a marketer’s arsenal, but it shouldn’t work in isolation or attempt to replace human intuition. While AI excels at identifying patterns, segmenting audiences, and predicting behaviours, it lacks the contextual understanding and creativity that human marketers bring to the table.
The most successful businesses use AI to support and enhance decision-making, leveraging AI-driven insights to inform strategy while still applying human oversight to ensure messaging remains relevant, brand-aligned, and contextually appropriate. AI should act as an accelerator, streamlining processes and offering real-time insights while leaving the strategic direction and creative execution in human hands.
Align AI with Business Goals
For AI-driven personalisation to succeed, it must be closely aligned with measurable business objectives. Many companies make the mistake of implementing AI without clearly defining what success looks like, leading to misaligned expectations and poor ROI. AI personalisation should contribute to tangible outcomes such as increased customer retention, higher conversion rates, and improved customer lifetime value.
Businesses should establish clear key performance indicators (KPIs) to track AI’s impact, measuring not just surface-level engagement metrics like click-through rates, but also long-term retention, revenue uplift, and customer satisfaction. By continuously refining AI models based on performance data, businesses can ensure that personalisation strategies remain effective and adaptable to changing customer behaviours.
Conclusion
AI is a powerful tool, but it’s not a shortcut to effective personalisation in digital marketing. Businesses that succeed with AI-driven personalisation prioritise data infrastructure first, ensuring their data is clean, integrated, and real-time before deploying AI models. Without a strong data foundation, even the most sophisticated AI solutions will fail to deliver the level of personalisation customers expect.
Before making further investments in AI and personalisation, businesses should assess their data readiness, align teams, and focus on integration. AI should always enhance personalisation strategies, not replace them. When combined with high-quality, structured data and human expertise, AI has the potential to elevate customer experiences, drive engagement, and deliver measurable business results.
Want to know if your data is ready for AI personalisation? Explore Plinc’s solutions for data-driven marketing personalisation and stay tuned for our upcoming report on predictive insights in customer segmentation.
Blog posts
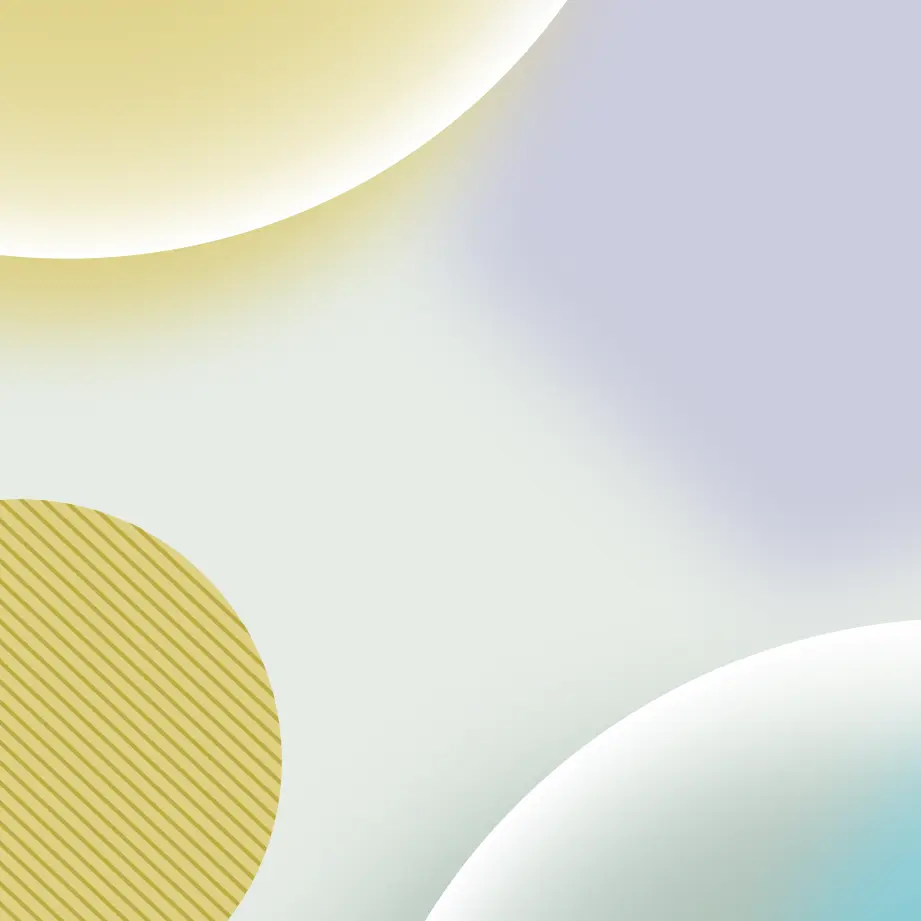
Become a Customer Economist: Why CRM Should Speak in Value, Not Volume
CRM is often rich in insight but fails to speak the business’s language. Reframing performance in terms of customer value is key to influencing strategy…
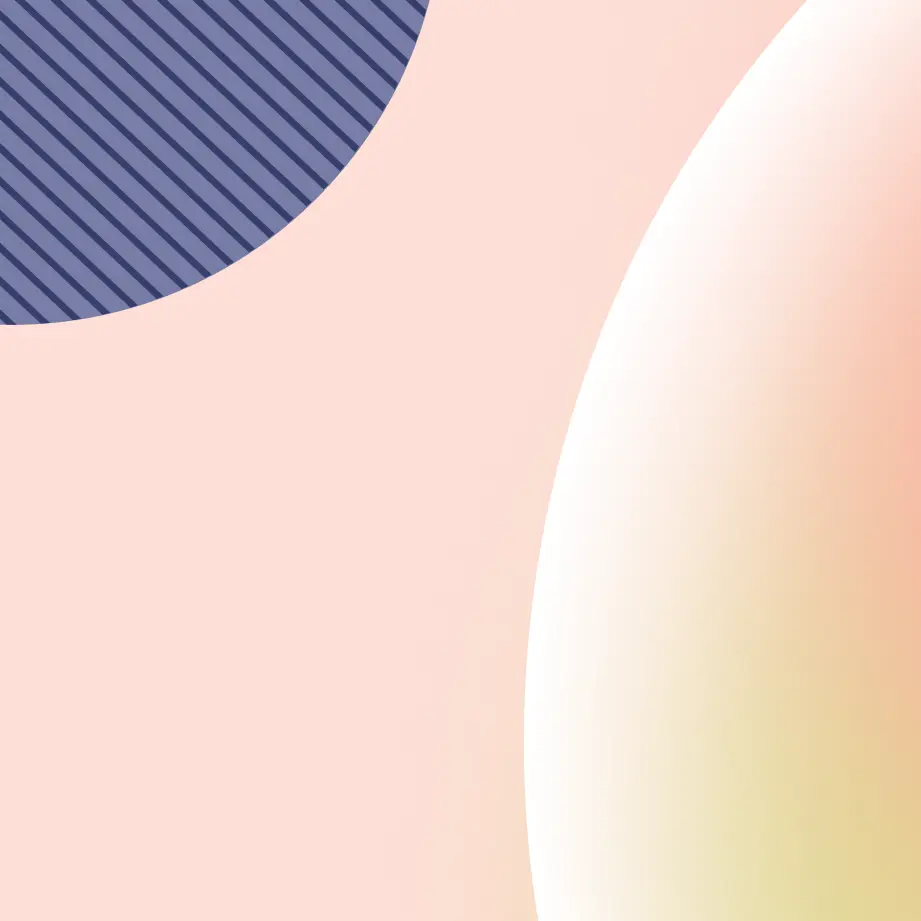
From Batch to Precision: Winning the Relevance War Without Burning Out Your Base
Batch campaigns create CRM fatigue fast. Shifting to precision targeting protects customer value, boosts engagement efficiency, and builds sustainable…
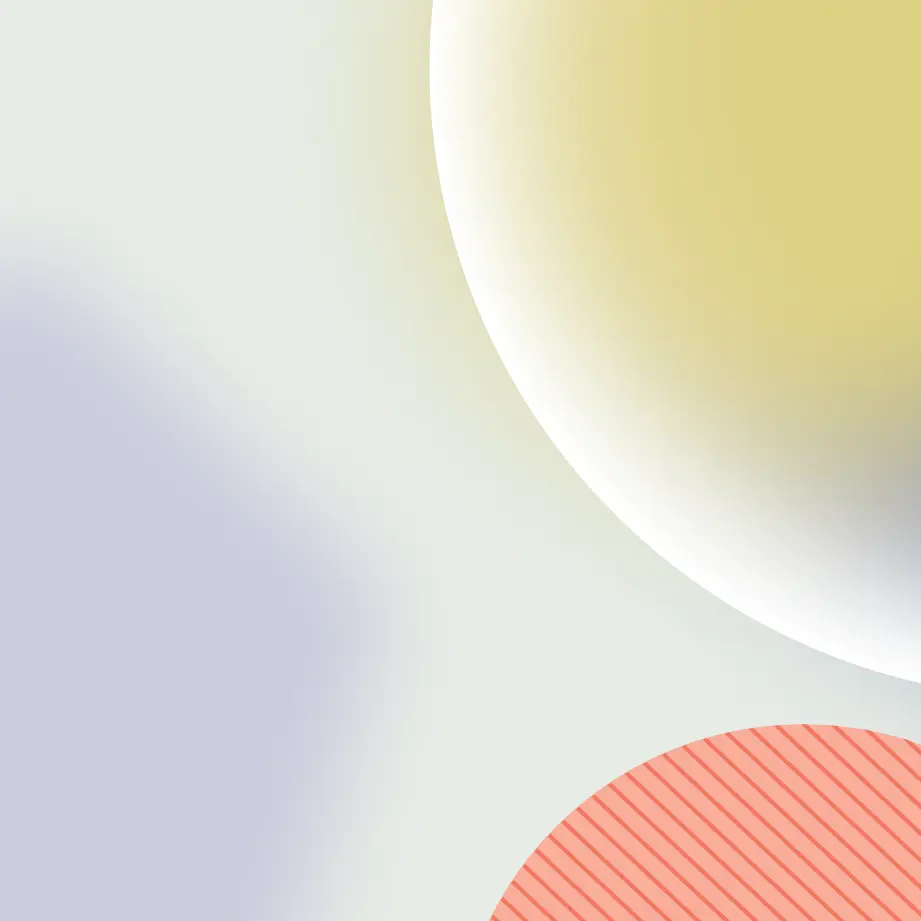
Prove It or Lose It: Why CRM Needs to Speak in Revenue, Not Reach
CRM teams are under pressure to prove commercial impact. Without uplift measurement and control groups, incremental revenue stays invisible and influence…
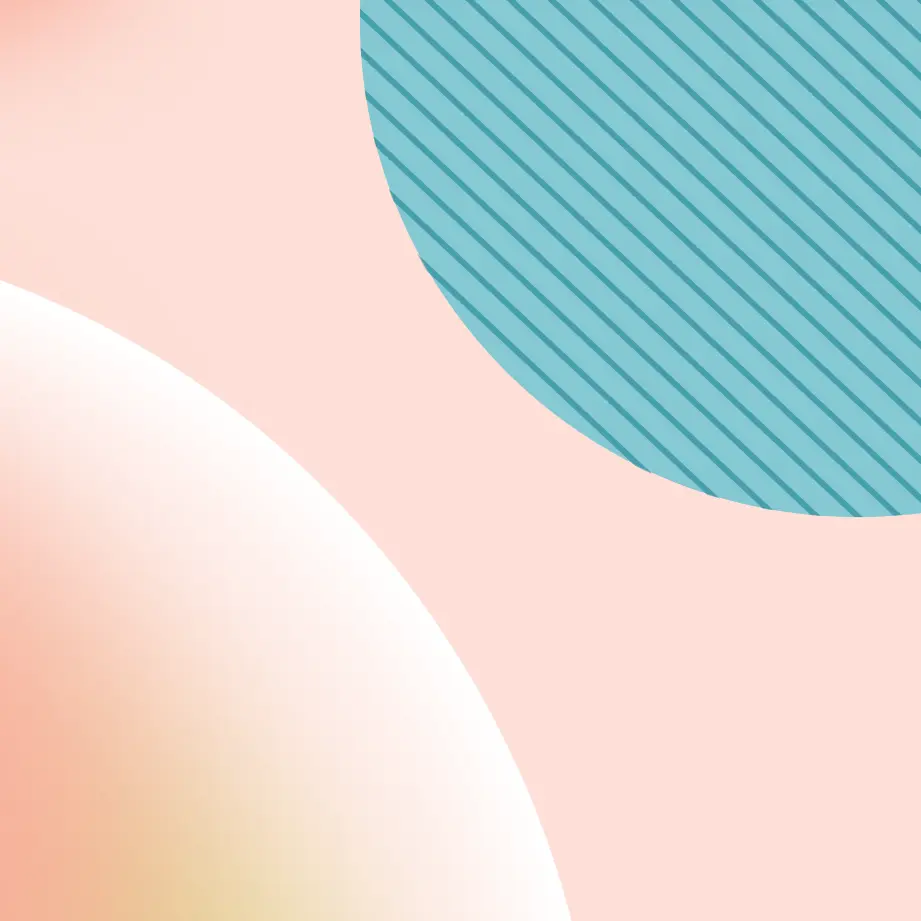
Sound the Alarm: Why CRM’s Data Problem Is a Strategic Problem
Inaccessible data continues to limit the impact of CRM. Yet when it’s reframed as a commercial issue rather than a technical one, it opens the door to…
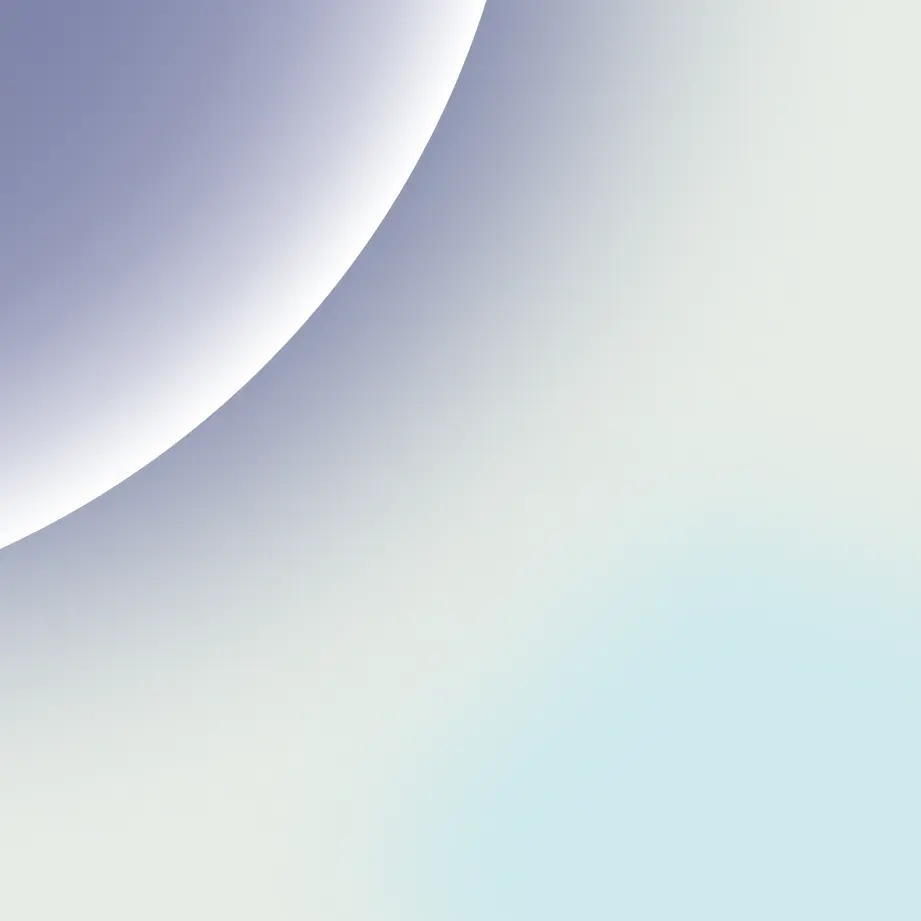
From Execution to Influence: Why It’s Time for CRM to Take the Lead
Introducing Plinc’s Strategic CRM Playbook: From Execution to Influence. Discover why CRM is poised to lead with insight, value, and influence in today’s…
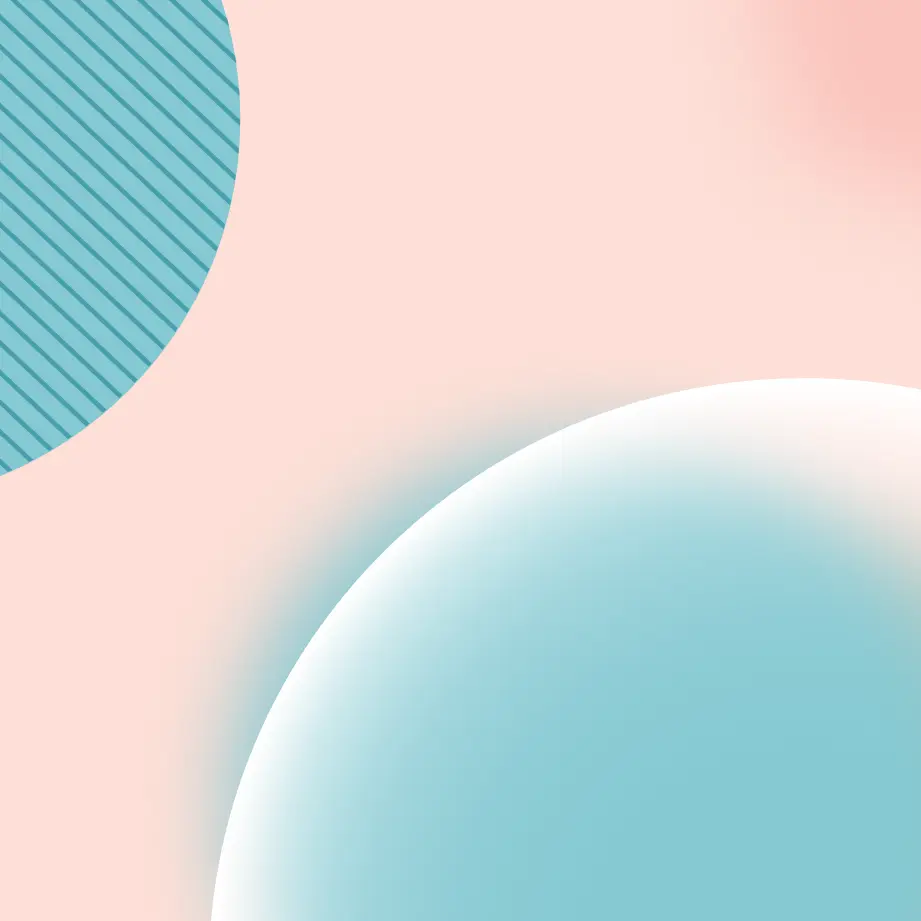
Event Debrief: Why Loyalty Isn’t About Points And Personalisation Isn’t About Tech
Insights from Plinc and DMA North’s breakfast briefing in Leeds, where senior CRM leaders shared what’s really working in loyalty, trading, and customer…